Introduction:
In the rapidly evolving world of artificial intelligence, Microsoft has unleashed a groundbreaking AI model known as Microsoft ORCA. This revolutionary model, inspired by the reasoning process of the formidable GPT-4, has captured the attention of researchers and developers worldwide. In this blog post, we will explore the ins and outs of ORCA, understanding its significance and the reasons behind its emergence.
1. Introduction to ORCA and its Significance in the AI Landscape:
Introduce ORCA as a cutting-edge AI model developed by Microsoft.
Highlight the anticipation and excitement surrounding ORCA’s release.
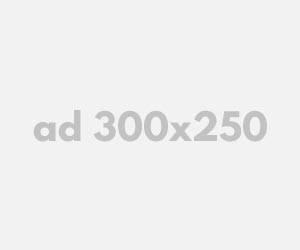
Explain the importance of ORCA’s breakthrough in the field of AI.
Mention the impact it is expected to have on the AI research community.
2. Brief Comparison between ORCA and GPT-4:
While GPT-4 stands as one of the most powerful language models, ORCA introduces a new paradigm by building upon its capabilities. Let’s take an in-depth look at the key points of comparison between ORCA and GPT-4, showcasing how ORCA surpasses its predecessor in certain aspects.
ORCA’s emphasis on accessibility, efficient resource utilization, enhanced reasoning, and transparency sets it apart from the larger GPT-4 model. By learning from complex explanation traces, ORCA acquires a deeper understanding of reasoning and problem-solving, providing valuable insights into its decision-making process. Additionally, ORCA’s stellar performance in various benchmarks demonstrates its ability to excel in tasks requiring comprehension, reasoning, and explanation generation.

In the next sections, we will explore how ORCA leverages explanation traces and its impressive performance on different benchmarks, further showcasing its superiority over other models.
Learning from Explanation Traces and Impressive Benchmark Performance:
One of the key differentiating factors of Microsoft ORCA is its ability to learn from complex explanation traces generated by GPT-4. These traces serve as a roadmap to GPT-4’s reasoning and problem-solving process, providing detailed insights into how the larger model arrives at its conclusions. ORCA leverages these explanation traces as valuable training data, enabling it to imitate and enhance its reasoning capabilities based on the knowledge gained.
By studying the intricate explanations, ORCA goes beyond simple imitation and generates its own explanations when faced with similar tasks. It compares these self-generated explanations with those of GPT-4, allowing for iterative improvements and fine-tuning. This unique approach sets ORCA apart from other smaller models, as it focuses not only on mimicking the larger model but also on understanding and replicating the reasoning process behind it.
The learning process of ORCA heavily relies on explanation traces obtained from a vast collection of tasks and instructions known as “FLAN 2022.” This extensive dataset covers a wide spectrum of subjects, enabling ORCA to gain insights and learn from diverse and intricate tasks. By sampling from FLAN 2022, ORCA acquires the ability to query GPT-4 for explanation traces, further expanding its understanding and problem-solving capabilities.
Various benchmarks is truly remarkable. It surpasses other open-source models of similar or even larger sizes, showcasing its enhanced reasoning, comprehension, and generative abilities. Let’s take a closer look at some of the benchmark performances:
1. Big Bench Hard (BBH): ORCA achieves an impressive accuracy score of 64, surpassing the performance of models like Vikuna 13B (30), Chat GPT (59), and even GPT-4 (62).
2. SuperGLUE (SG): ORCA maintains an average score of 86, outperforming Vikuna 13B (81), TextDaVinci 003 (83), and Chat GPT (84), and closely matching the performance of GPT-4 (88).
3. CNN Daily Mail (CDM): ORCA achieves a Rugel score of 41, outshining Vikuna 13B (38), TextDaVinci 003 (39), Chat GPT (40), and approaching the performance level of GPT-4 (42).
4. COCO Captions (CC): ORCA secures a remarkable CIDEr score of 120, surpassing Vikuna 13B (113), TextDaVinci 003 (115), Chat GPT (117), and demonstrating competitiveness with GPT-4 (119).
These benchmark results demonstrate the superior performance of ORCA in tasks that require reasoning, comprehension, and generative capabilities. Despite its smaller size, ORCA consistently outperforms other models, even rivaling the performance of larger models like GPT-4.
Broader Implications of ORCA’s Success:
It holds significant implications for the future of AI research and development. Here are some key points to consider:
Enhancing AI Intelligence: Learning from explanations, as demonstrated by Microsoft ORCA, offers a powerful approach to boost AI intelligence and performance. By understanding and replicating the reasoning process of larger models, smaller models like ORCA can achieve superior reasoning skills, resulting in more accurate and relevant outputs.
Transparency and Interpretability: ORCA’s ability to generate detailed explanation traces contributes to the transparency and interpretability of AI models. This transparency builds trust and enables users to validate the model’s reasoning process. It also opens avenues for further research in understanding how AI models make decisions.
Accessible and Efficient AI: ORCA represents a step toward more accessible and efficient AI models. Its smaller size and ability to deliver impressive performance make it more attainable for researchers and developers. This accessibility allows a wider range of individuals to leverage the power of advanced AI models without the constraints of extensive resources.
Potential of Explanation-Based Learning: ORCA’s success highlights the potential of explanation-based learning in AI. By leveraging explanations, models can gain valuable insights, refine their reasoning abilities, and expand their knowledge base. This opens up new possibilities for developing AI models that are both highly capable and transparent.
3. Exploring the Motivations behind Developing a Smaller, More Specialized Model:
Discuss the motivations that led to the creation.
Explain the challenges associated with larger models, such as high costs, computing resource requirements, and limited accessibility.
Highlight the growing interest in developing smaller models that maintain high performance while being more efficient and accessible.
Introduce ORCA as an answer to these challenges, with its focus on accessibility, efficiency, and specialization.
By understanding the significance of ORCA and the motivations behind its development, we can delve deeper into its architecture, capabilities, and the impact it is poised to make in the realm of artificial intelligence.